Where Do Location Data Services Stand in a Privacy-Oriented World?
Foursquare’s Vikram Gundeti discusses navigating a privacy-first approach to providing information that comes from aggregate data related to movements.
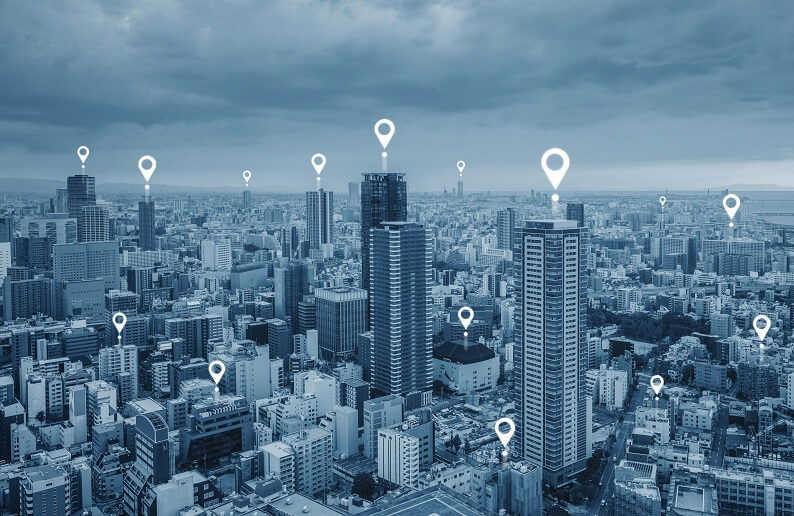
Mounting scrutiny of how personal data is used continues to create new considerations for organizations that have relied on finding, tracking, and targeting individuals as part of their operational strategy. Location-based services that sought to deliver marketing campaigns through mobile devices, for example, might get some pushback from a public that now seeks stricter awareness and control over what is known and shared about them.
There may be ways for organizations to continue to derive location-centric understandings of the customers while also respecting data privacy.
Vikram Gundeti, vice president and distinguished engineer with Foursquare, spoke with InformationWeek about being privacy-first while working in location services.
One of the driving conversations we’re having these days is around the increased awareness and questions about data privacy. When it comes to location intelligence, how can you work from a privacy-first perspective with something that is trying to -- by its nature -- track you?
I’ll start with a very sensitive category and an example where privacy becomes important: Advertising. And if you look at the 2010s, a lot of the focus in advertising has been around building this identity graph, which is to organize all your information around individuals and use that information to target people wherever they are.
So, where we see an opportunity in terms of pivoting that, one is the regulation landscape and increased awareness of sensitivity or privacy-related aspects -- getting individualized information is going to be difficult and even if you get that individualized information, how do you use it?
You need to be very careful in terms of what you can use it for. Regulation is one aspect, but if I’m using a product, I want to be making sure that my data is not used in a way that I’m not aware of. Where we are pivoting, where we think the promise of the Foursquare Graph is, instead of organizing all our assets around people, we are organizing around location.
And location as in, you can go from different granularities and that’s where the H3 element is going to be. H3 is the hexagonal grid system. If you look at location as an entity, one of the big realizations that led to this is there is no one unit that’s the standard unit of location.
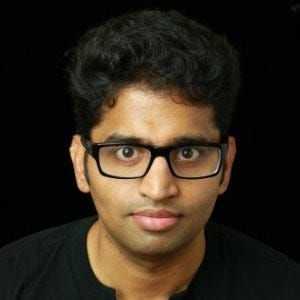
Vikram Gundeti, Foursquare
You have points; you have neighborhoods; you have counties and all that. What we found was if we organize all our information around these H3 cells, or indirectly through H3 cells, we can go from any granularity of locations, whether it is a place or whether it is a neighborhood or a city or whatever.
Now, how that changes the pattern is instead of asking, “Hey, find me all the people who are sports enthusiasts in the age group 35 to 40,” and then today you get the advertising IDs of those people, and you go and use that information to target them on different advertising platforms.
What we are trying to do is take that information and build location profiles. Foursquare by virtue of its movement data, which are much more robust than traffic or any other data, we know where people are spending time in the aggregate.
The goal of the advertiser is to reach a certain population, so instead of trying to go reach these specific people, what we can provide now is, “Hey, there is a high probability of reaching this audience if you target them at this location at this time.” And that opens up a lot of avenues because you don’t need individualized information. You’re trying to understand aggregate patterns. It even opens up new use cases for us. If you have a digital billboard, it’s at an intersection and you know this intersection has a mall that gets busy around this time with this kind of demographic. You can change the kind of ads you display to that kind of audience. So that’s where I think the pivot from using the individual as an entity to location as an entity becomes very crucial in our strategy.
Can you share the history or a timeline for Graph tech support?
If you look at the genesis of the Graph, internally for us, it started as an engineering problem. There are two lenses from which we look at it. One is if we take each of our individual products like places or movements, we found opportunities where we can make them better where we can join them with other datasets in general.
If I know a place has a Lat Long that is falling on a road, it’s likely that is incorrect. We will use techniques to snap them to the closest building and fix those kinds of things. Similarly, our movements data can benefit from some of the insights we derive while generating the places. For instance, our movements data can capture information about, “Hey, what is the standard entry point or drop off point to this place?” That is important for companies like Uber.
We had very vertical silos of these product stacks because of their revolution and our acquisitions, which made it difficult for us to do this data sharing.
The first thing was it was for our own benefit we thought of organizing data, and we have close to 35 to 40 different kinds of assets that can be organized around people, locations, events happening at locations, and a bunch of different things. That helped us improve our own products. That was the first impetus for this.
The second thing is we started looking at is how our customers are consuming data and what is the persona of the customers. We found that it was heavily skewed towards these big companies which had investments in handling this kind of data, and they had to do significant investment before they are able to reap benefits from the data. Our thesis was if you join all these data beforehand and provide more nuanced insights around two dimensions -- one is the space dimension and the time dimension -- I can ask questions around that.
An example I always use is, you can use a Yelp star rating to go to a restaurant, or you could say, “Where do people who live and work in this location go for lunch,” which is a higher quality signal than a star rating. Our goal was to reduce the time to insights to get to the actual business problem our customers are trying to support.
If someone is trying to locate a store, they say, there are three different malls in the city. Here’s the average dwell time. Here is the age group and income level of the people visiting this mall. We make sure that there is a certain volume before we infer a pattern so that it doesn’t recommend individuals.
Are there other aspects of having a privacy-first approach that have not been part of other enterprises’ conversations or have been slow to become part of the conversation? Are there other ways, additional things that can be done for that privacy-first approach?
I think one of the things is that when enterprises are handling -- if you are a mobile app developer, building location-centric experience becomes so crucial. Your context around where, whether it is food delivery, and all that. Retail apps, gig economy kind of apps, and all that require location as a key element.
From an understanding of a safety perspective, if it’s a dating app, you want to understand where you are going if they’re planning a meet up, whether that location is safe or not from a different perspective.
There is a huge heavy lift now with all the regulation coming along in terms of handling the data and making sure you are compliant with the different regulations.
What Foursquare has done over the years because it has been early on in the location game, has built this set of tools -- whether it does not track, access your data, or delete your data -- whether it is our first-party data, second-party data we acquire through our SDK partners, or third-party data we acquired from specific vendors, we made sure they’re compliant with all these things. They get explicit consent from our customers.
What other factors are influencing the future development and evolution of geospatial data? Right now, every other thing is about AI and generative AI. Is there anything specific to geospatial data happening there?
I think the one thing with generative AI and all that is the recommendations will be as good as the foundational data, and they have a tendency to hallucinate a lot. I think focusing on the key data modes is going to be important even in this world of generative AI because that part is being commoditized at an astronomical pace with the open-source models and all that. It’s less about the interface now. It’s more about what data, what unique assets you are having, what kind of relationships do you have represented in your data model? Our goal is to train a model so that we can derive insights. Some of the things we do more declaratively today is when we provide a recommendation for a place in our app, we also provide a justification saying that we are giving you this because your friend visited it, or this is a food you said you liked at a different place.
Those kinds of justifications will also be made possible through this data and applying the AI techniques.
What to Read Next:
Congressional Subcommittee Holds Hearing on Data Privacy Policy
Pivotal Moments In Data Privacy History
Will Your Company Be Fined in the New Data Privacy Landscape?
About the Author(s)
You May Also Like
How to Amplify DevOps with DevSecOps
May 22, 2024Generative AI: Use Cases and Risks in 2024
May 29, 2024Smart Service Management
June 4, 2024